Space-Time Tree Ensemble for Action Recognition
We explore ensembles of hierarchical spatio-temporal trees, discovered directly from training data, to model these structures for action recognition.
June 7, 2015
IEEE Conference on Computer Vision Pattern Recognition (CVPR) 2015
Authors
Shugao Ma (Boston University)
Leonid Sigal (Disney Research)
Stan Sclaroff (Boston University)
Space-Time Tree Ensemble for Action Recognition
Human actions are, inherently, structured patterns of body movements. We explore ensembles of Hierarchical spatio-temporal trees, discovered directly from training data, to model these structures for action recognition. The Hierarchical spatio-temporal trees provide a robust midlevel representation for actions. However, discovery of frequent and discriminative tree structures is challenging due to the exponential search space, particularly if one allows partial matching. We address this by first building a concise action vocabulary via discriminative clustering. Using the action vocabulary, we then utilize tree mining with subsequent tree clustering and ranking to select a compact set of highly discriminative tree patterns. We show that these tree patterns, alone, or in combination with shorter patterns (action words and pairwise patterns) achieve state-of-the-art performance on two challenging datasets: UCF Sports and HighFive. Moreover, trees learned on HighFive are used in recognizing two action classes in a different dataset, Hollywood3D, demonstrating the potential for cross-dataset generality of the trees our approach discovers.
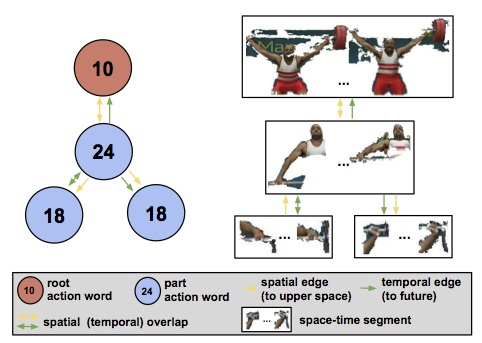