Harnessing Object and Scene Semantics for Large-Scale Video Understanding
We propose a novel object- and scene-based semantic fusion network and representation.
June 27, 2016
IEEE Conference on Computer Vision Pattern Recognition (CVPR) 2016
Authors
Zuxuan Wu (Fudan University)
Yanwei Fu (Disney Research)
Yu-Gang Jiang (Fudan University)
Leonid Sigal (Disney Research)
Large-scale action recognition and video categorization are important problems in computer vision. To address these problems, we propose a novel object- and scene-based semantic fusion network and representation. Our semantic fusion network combines three streams of information using a three-layer neural network: (i) frame-based low-level CNN features, (ii) object features from a state-of-the-art large-scale CNN object-detector trained to recognize 20K classes, and (iii) scene features from a state-of-the-art CNN scene-detector trained to recognize 205 scenes. The trained network achieves improvements in supervised activity and video categorization in two complex large-scale datasets – ActivityNet and FCVID, respectively. Further, by examining and back propagating information through the fusion network, semantic relationships (correlations) between video classes and objects/scenes can be discovered. These video class-object/video class-scene relationships can, in turn, be used as semantic representation for the video classes themselves. We illustrate effectiveness of this semantic representation through experiments on zero-shot action/video classification and clustering.
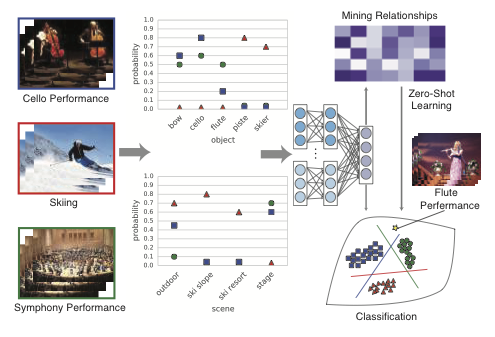