Adaptive Polynomial Rendering
We propose a new adaptive rendering method to improve the performance of Monte Carlo ray tracing, by reducing noise contained in rendered images while preserving high-frequency edges.
July 11, 2016
ACM SIGGRAPH 2016
Authors
Bochang Moon (Disney Research)
Steven McDonagh (Disney Research)
Kenny Mitchell (Disney Research/Edinburgh Napier University)
Markus Gross (Disney Research/ETH Zürich)
Adaptive Polynomial Rendering
In this paper, we propose a new adaptive rendering method to improve the performance of Monte Carlo ray tracing, by reducing noise contained in rendered images while preserving high-frequency edges. Our method locally approximates an image with polynomial functions and the optimal order of each polynomial function is estimated so that our reconstruction error can be minimized. To robustly estimate the optimal order, we propose a multi-stage error estimation process that iteratively estimates our reconstruction error. In addition, we present an energy-preserving outlier removal technique to remove spike noise without causing noticeable energy loss in our reconstruction result. Also, we adaptively allocate additional ray samples to high error regions guided by our error estimation. We demonstrate that our approach outperforms state-of-the-art methods by controlling the tradeoff between reconstruction bias and variance through locally defining our polynomial order, even without need for filtering bandwidth optimization, the common approach of other recent methods.
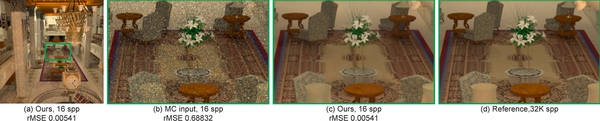