A Task-level iterative learning control algorithm for accurate tracking in manipulators with modeling errors and stringent joint position limits
We present an iterative learning control algorithm for accurate task space tracking of kinematically redundant robots with stringent joint position limits and kinematic modeling errors.
October 12, 2016
Dynamic Systems and Control Conference 2016
Authors
Pranav Bhounsule (University of Texas San Antonio)
Abhishek Bapat (University of Texas San Antonio)
Katsu Yamane (Disney Research)
The iterative learning control update rule is in the task space and consists of adding a correction to the desired end-effector pose based on the tracking error. The new desired end-effector pose is then fed to an inverse kinematics solver that uses the redundancy of the robot to compute feasible joint positions. We discuss the stability, the rate of convergence and the sensitivity to learning gain for our algorithm using quasi-static motion examples. The efficacy of the algorithm is demonstrated on a simulated four link manipulator with joint position limits that learns the modeling error to draw the figure eight in 4 trials.
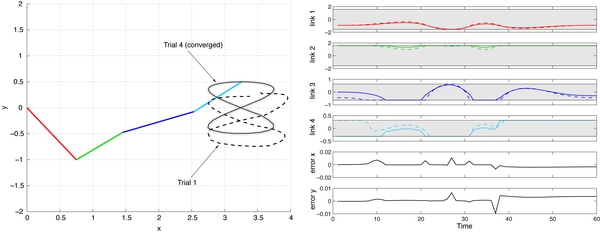