Data-Driven Ghosting using Deep Imitation Learning
Motivated by the original “ghosting” work, we showcase an automatic “data-driven ghosting” method using advanced machine learning methodologies applied to a season’s worth of tracking data from a recent professional league in soccer.
March 3, 2017
MIT Sloan Sports Analytics Conference 2017
Authors
Hoang M. Le (California Institute of Technology)
Peter Carr (Disney Research)
Yisong Yue (California of Technology)
Patrick Lucey (STATS LLC)
Data-Driven Ghosting using Deep Imitation Learning
An example of our approach is depicted in Figure 1 which illustrates a scoring chance that Fulham (red) created against Swansea (blue). Suppose we are interested in analyzing the defensive movements of Swansea. It might be useful to visualize what the team actually did compared to what a typical team in the league might have done. Using our approach, we are able to generate the defensive motion pattern of the “league average” team, which interestingly results in a similar expected goal value (69.1% for Swansea and 71.8% for the “league average” ghosts — to fully appreciate the insights revealed by data-driven ghosting, we urge the readers to view the supplemental video.
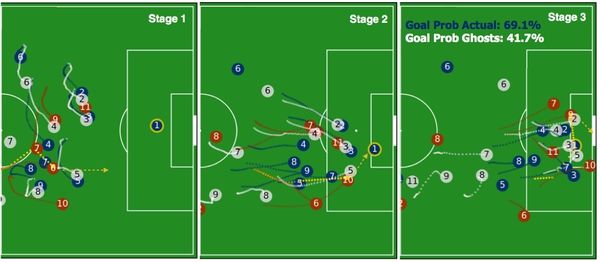