Learning and Reusing Dialog for Repeated Interactions with a Situated Social Agent
This paper introduces an embodied agent that self-authors its own dialog for social chat.
August 27, 2017
International Conference on Intelligent Virtual Agents (IVA) 2017
Authors
James Kennedy (Disney Research)
Iolanda Leite (Disney Research, KTH Royal Institute of Technology)
André Pereira (Disney Research)
Ming Sun (Disney Research)
Boyang Li (Disney Research)
Rishub Jain (Disney Research/Carnegie Mellon University)
Ricson Cheng (Disney Research/Carnegie Mellon University)
Eli Pincus (Disney Research/USC Institute for Creative Technologies)
Elizabeth J. Carter (Disney Research)
Jill Fain Lehman (Disney Research)
Learning and Reusing Dialog for Repeated Interactions with a Situated Social Agent
Content authoring for conversations is a limiting factor in creating verbal interactions with intelligent virtual agents. Building on techniques utilizing semi-situated learning in an incremental crowd-working pipeline, this paper introduces an embodied agent that self-authors its own dialog for social chat. In particular, the autonomous use of crowd- workers is supplemented with a generalization method that borrows and assesses the validity of dialog across conversational states. We argue that the approach offers a community-focused tailoring of dialog responses that is not available in approaches that rely solely on statistical methods across big data. We demonstrate the advantages that this can bring to interactions through data collected from 486 conversations between a situated social agent and 22 users during a 3 week long evaluation period.
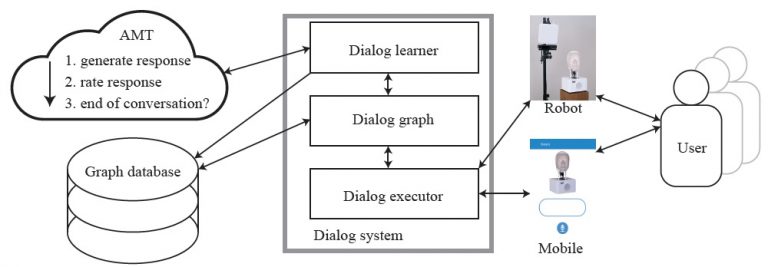