Towards Edge-Aware Spatio-Temporal Filtering in Real-Time
We propose an efficient STEA filtering pipeline based on the recently proposed permeability filter (PF) which offers high quality and halo reduction capabilities.
October 2, 2017
IEEE Transactions on Image Processing 2017
Authors
Michael Schaffner (Disney Research/ETH Joint PhD)
Florian Scheidegger (Disney Research/ETH Joint M.Sc.)
Lukas Cavigelli (ETH Zurich)
Hubert Kaeslin (ETH Zurich)
Luca Benini (ETH Zurich)
Aljosa Smolic (Trinity College Dublin)
Towards Edge-Aware Spatio-Temporal Filtering in Real-Time
Spatio-temporal edge-aware (STEA) filtering methods have recently received increased attention due to their ability to efficiently solve or approximate important imagedomain problems in a temporally consistent manner – which is a crucial property for video-processing applications. However, existing STEA methods are currently unsuited for real-time, embedded stream-processing settings due to their high processing latency, large memory and bandwidth requirements,and the need for accurate optical flow to enable filtering along motion paths. To this end, we propose an efficient STEA filtering pipeline based on the recently proposed permeability filter (PF) which offers high quality and halo reduction capabilities. Using mathematical properties of the PF, we reformulate its temporal extension as a causal, non-linear infinite impulse response filter which can be efficiently evaluated due to its incremental nature. We bootstrap our own accurate flow using the PF and its temporal extension by interpolating a quasi-dense nearest neighbor field obtained with an improved PatchMatch algorithm, which employs binarized octal orientation maps (BOOM) descriptors to find correspondences among subsequent frames. Our method is able to create temporally consistent results for a variety of applications such as optical flow estimation, sparse data upsampling, visual saliency computation and disparity estimation. We benchmark our optical flow estimation on the MPI Sintel dataset, where we currently achieve a Pareto optimal quality-efficiency tradeoff with an average endpoint error of 7.68 at 0.59s single-core execution time on a recent desktop machine.
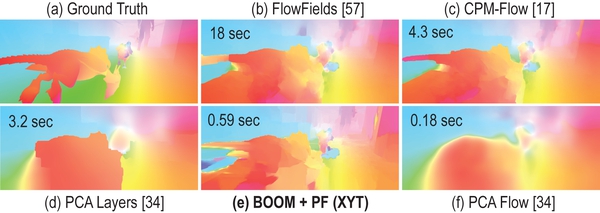