Robust Image Denoising using Kernel Predicting Networks
We present a new method for designing high quality denoisers that are robust to varying noise characteristics of input images.
May 3, 2021
Eurographics 2021
Authors
Zhilin Cai (DisneyResearch|Studios/ETH Joint M.Sc.)
Yang Zhang (DisneyResearch|Studios)
Marco Manzi (DisneyResearch|Studios)
Cengiz Oztireli (DisneyResearch|Studios)
Markus Gross (DisneyResearch|Studios/ETH Zurich)
Tunc Aydin (DisneyResearch|Studios)
We present a new method for designing high quality denoisers that are robust to varying noise characteristics of input images. Instead of taking a conventional blind denoising approach or relying on explicit noise parameter estimation networks as well as invertible camera imaging pipeline models, we propose a two-stage model that first processes an input image with a small set of specialized denoisers, and then passes the resulting intermediate denoised images to a kernel predicting network that estimates per-pixel denoising kernels. We demonstrate that our approach achieves robustness to noise parameters at a level that exceeds comparable blind denoisers, while also coming close to state-of-the-art denoising quality for camera sensor noise.
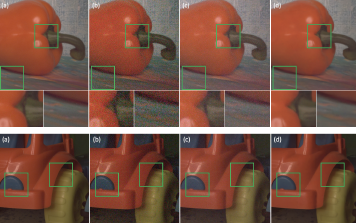