Implicit Neural Representation for Physics-driven Actuated Soft Bodies
We apply the method to volumetric soft bodies, human poses, and facial expressions, demonstrating artist-friendly properties.
July 24, 2022
ACM SIGGRAPH 2022
Authors
Lingchen Yang (ETH Zürich)
Byungsoo Kim (ETH Zürich)
Gaspard Zoss (DisneyResearch|Studios/ETH Joint PhD)
Baran Gözcü (ETH Zürich)
Markus Gross (DisneyResearch|Studios/ETH)
Barbara Solenthaler (ETH Zürich)
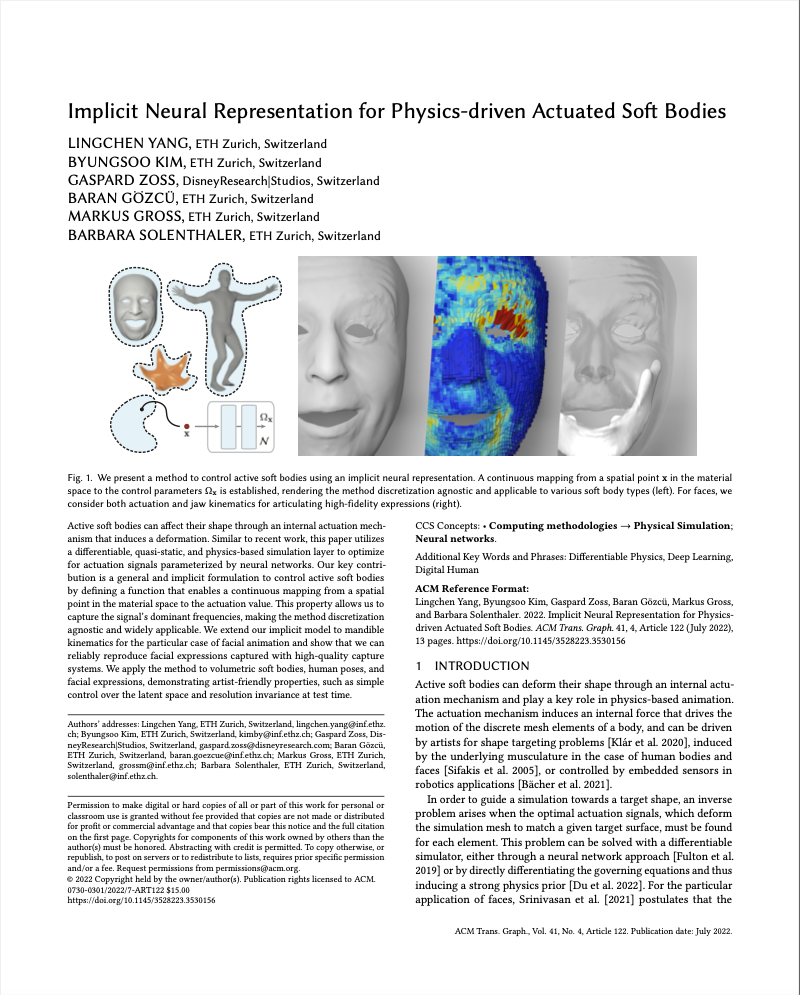
Implicit Neural Representation for Physics-driven Actuated Soft Bodies
Active soft bodies can affect their shape through an internal actuation mechanism that induces a deformation. Similar to recent work, this paper utilizes a differentiable, quasi-static, and physics-based simulation layer to optimize for actuation signals parameterized by neural networks. Our key contribution is a general and implicit formulation to control active soft bodies by defining a function that enables a continuous mapping from a spatial point in the material space to the actuation value. This property allows us to capture the signal’s dominant frequencies, making the method discretization agnostic and widely applicable. We extend our implicit model to mandible kinematics for the particular case of facial animation and show that we can reliably reproduce facial expressions captured with high-quality capture systems. We apply the method to volumetric soft bodies, human poses, and facial expressions, demonstrating artist-friendly properties, such as simple control over the latent space and resolution invariance at test time.
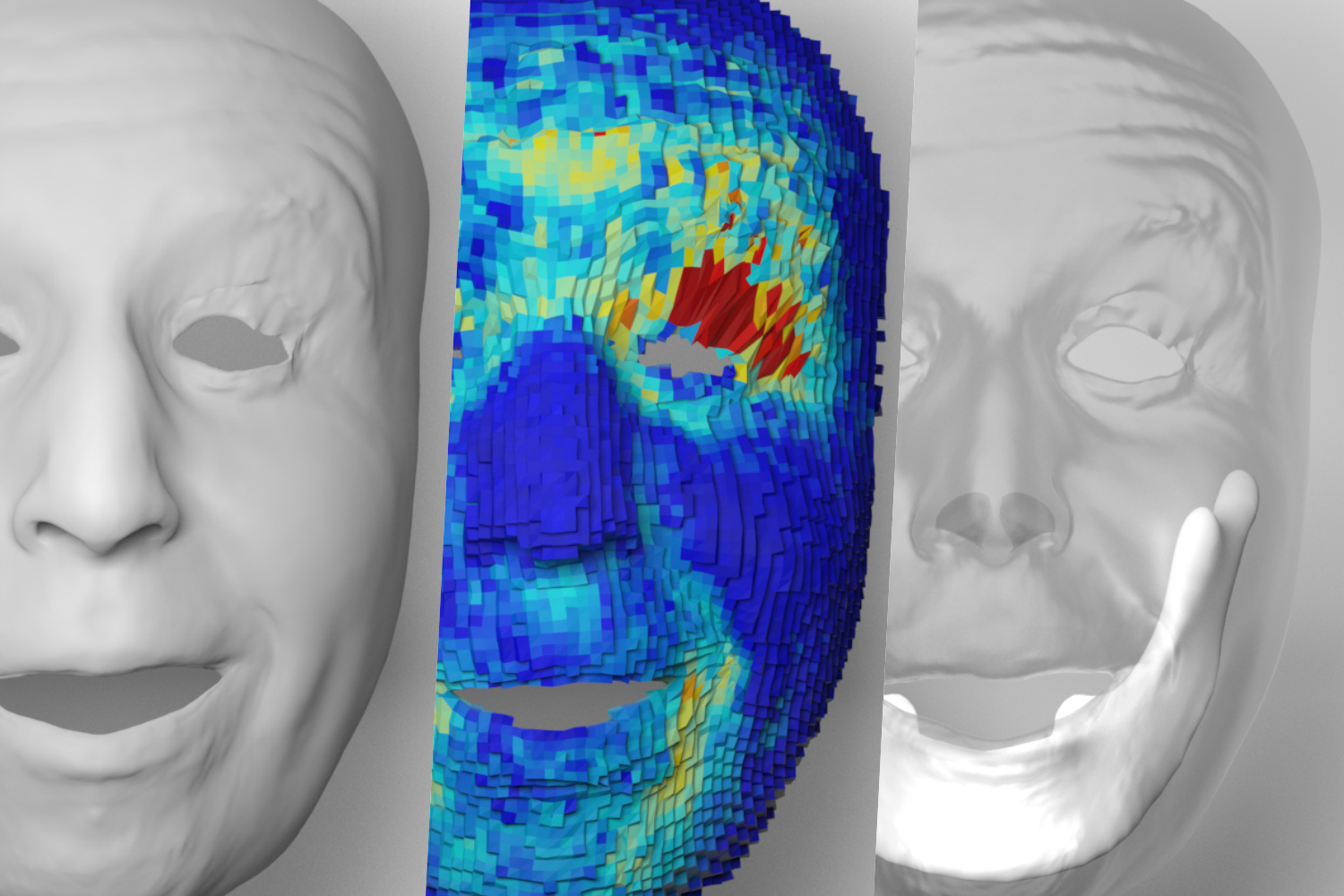