TempFormer: Temporally Consistent Transformer for Video Denoising
We propose an efficient hybrid Transformer-based model, TempFormer, which composes SpatioTemporal Transformer Blocks (STTB) and 3D convolutional layers.
October 11, 2022
European Conference on Computer Vision (ECCV) (2022)
Authors
Mingyang Song (ETH Zürich)
Yang Zhang (DisneyResearch|Studios)
Tunç O. Aydın (DisneyResearch|Studios)
Video denoising is a low-level vision task that aims to restore high-quality videos from noisy content. Vision Transformer (ViT) is a new machine learning architecture that has shown promising performance on both high-level and low-level image tasks, e.g., object detection, classification, and image restoration in the past year. In this paper, we propose a modified ViT architecture for video processing tasks, introducing a new training strategy and loss function to enhance temporal consistency without compromising spatial quality. Specifically, we propose an efficient hybrid Transformer-based model, TempFormer, which composes SpatioTemporal Transformer Blocks (STTB) and 3D convolutional layers. The proposed STTB learns the temporal information between neighboring frames implicitly by utilizing the proposed Joint Spatio-Temporal Mixer module for attention calculation and feature aggregation in each ViT block. Moreover, existing methods suffer from temporal inconsistency artifacts that are problematic in practical cases and distracting to the viewers. We propose a sliding block strategy with recurrent architecture, and use a new loss term, Overlap Loss, to alleviate the flickering between adjacent frames. Our method produces state-of-the-art spatio-temporal denoising quality with significantly improved temporal coherency and requires less computational resources to achieve comparable denoising quality with competing methods.
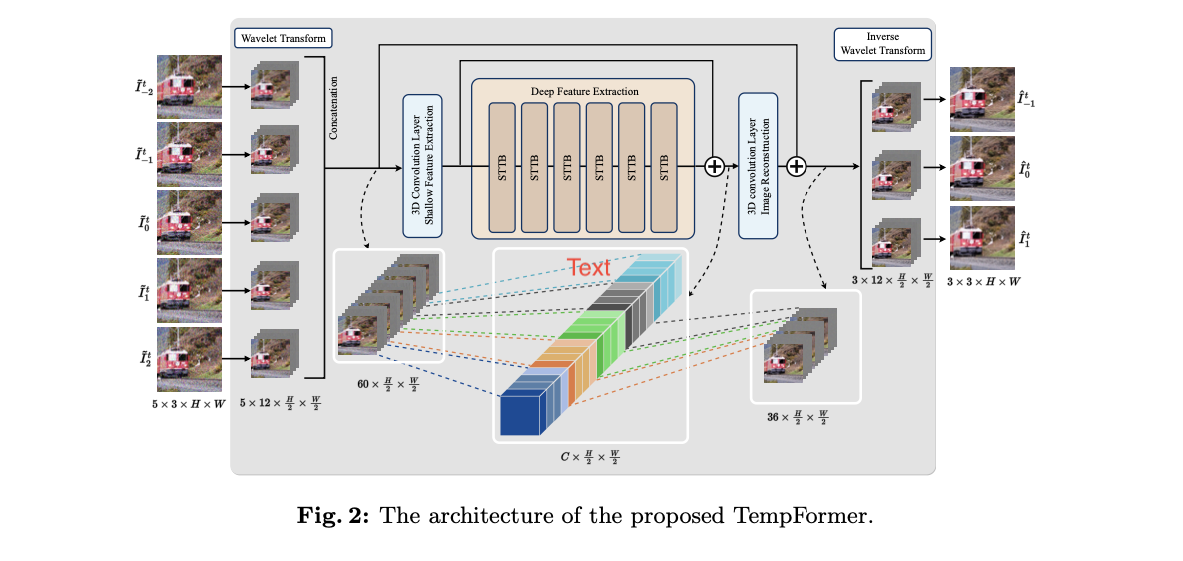