Controllable Inversion of Black-Box Face Recognition Models via Diffusion
We tackle the challenging task of inverting the latent space of pre-trained face recognition models without full model access (i.e. black-box setting). Our method, the identity denoising diffusion probabilistic model (ID3PM), leverages the stochastic nature of the denoising diffusion process to produce high-quality, identity-preserving face images with various backgrounds, lighting, poses, and expressions.
Authors
Manuel Kansy (DisneyResearch|Studios/ETH Joint PhD)
Anton Rael (ETH Zurich)
Graziana Mignone (DisneyResearch|Studios)
Jacek Naruniec (DisneyResearch|Studios)
Christopher Schroers (DisneyResearch|Studios)
Markus Gross (DisneyResearch|Studios /ETH Zurich)
Romann M. Weber (DisneyResearch|Studios)
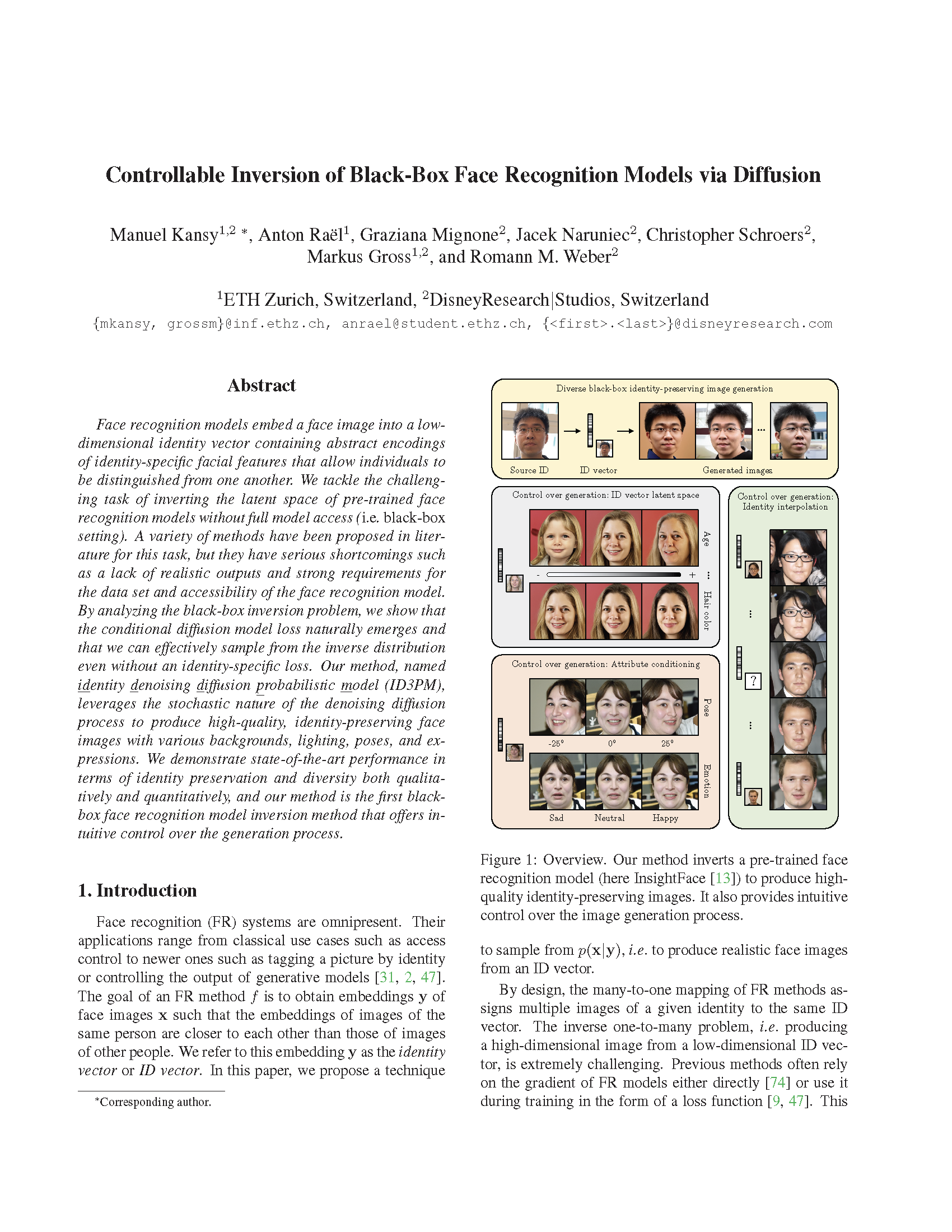
Controllable Inversion of Black-Box Face Recognition Models via Diffusion
Face recognition models embed a face image into a low-dimensional identity vector containing abstract encodings of identity-specific facial features that allow individuals to be distinguished from one another. We tackle the challenging task of inverting the latent space of pre-trained face recognition models without full model access (i.e. black-box setting). A variety of methods have been proposed in literature for this task, but they have serious shortcomings, such as a lack of realistic outputs and strong requirements for the data set, and accessibility of the face recognition model. By analyzing the black-box inversion problem, we show that the conditional diffusion model loss naturally emerges and that we can effectively sample from the inverse distribution even without an identity-specific loss. Our method, named identity denoising diffusion probabilistic model (ID3PM), leverages the stochastic nature of the denoising diffusion process to produce high-quality, identity-preserving face images with various backgrounds, lighting, poses, and expressions. We demonstrate state-of-the-art performance in terms of identity preservation and diversity both qualitatively and quantitatively, and our method is the first black-box face recognition model inversion method that offers intuitive control over the generation process.
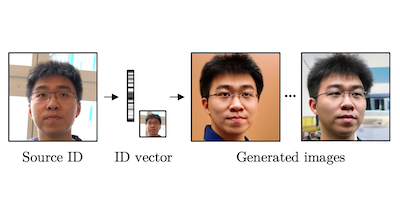