Combining Frame and GOP Embeddings for Neural Video Representation
In this paper, we propose T-NeRV, a hybrid video INR that combines frame-specific embeddings with GOP-specific features, providing a lever for content-specific fine-tuning.
June 17, 2024
CVPR (2024)
Authors
Jens Eirik Saethre (DisneyResearch|Studios/ETH Zurich)
Roberto Azevedo (DisneyResearch|Studios)
Christopher Schroers (DisneyResearch|Studios)
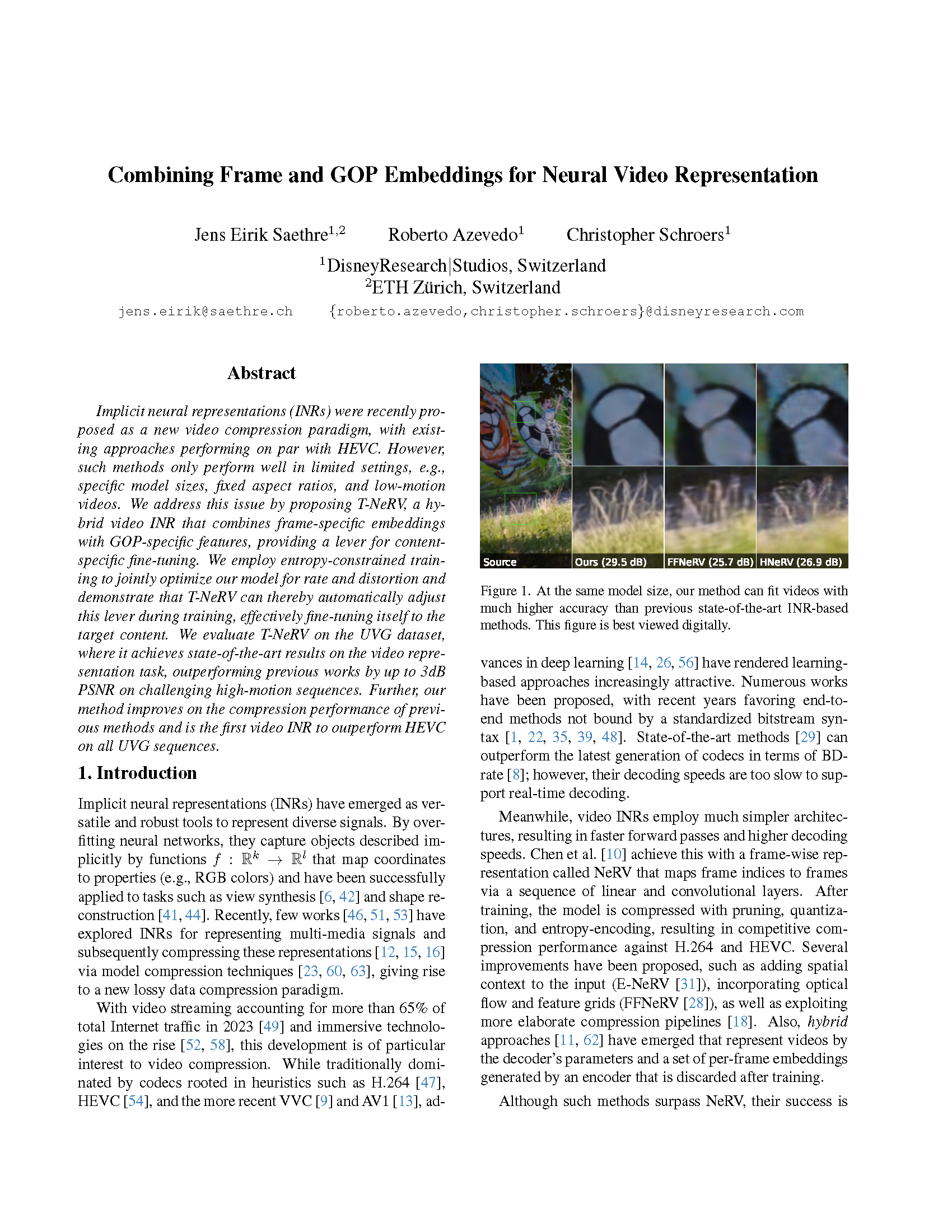
Combining Frame and GOP Embeddings for Neural Video Representation
Implicit neural representations (INRs) were recently proposed as a new video compression paradigm, with existing approaches performing on par with HEVC. However, such methods only perform well in limited settings, e.g., specific model sizes, fixed aspect ratios, and low-motion videos. We address this issue by proposing T-NeRV, a hybrid video INR that combines frame-specific embeddings with GOP-specific features, providing a lever for content-specific fine-tuning. We employ entropy-constrained training to jointly optimize our model for rate and distortion and demonstrate that T-NeRV can thereby automatically adjust this lever during training, effectively fine-tuning itself to the target content. We evaluate T-NeRV on the UVG dataset, where it achieves state-of-the-art results on the video representation task, outperforming previous works by up to 3dB PSNR on challenging high-motion sequences. Further, our method improves on the compression performance of previous methods and is the first video INR to outperform HEVC on all UVG sequences.
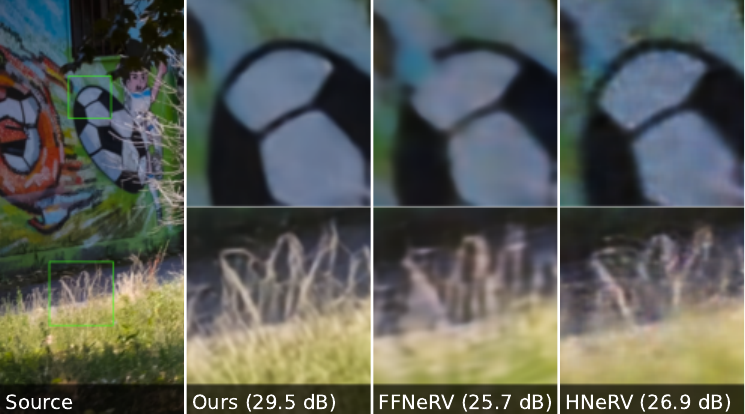