Deep Spatial Pyramid for Person Re-identification
We introduce a spatial pyramid-like structure to the image and learn CNNs for image sub-regions at different scales.
August 30, 2017
The 14th IEEE International Conference on Advanced Video and Signal-Based Surveillance (AVSS) 2017
Authors
Slawomir Bak (Disney Research)
Peter Carr (Disney Research)
Deep Spatial Pyramid for Person Re-identification
Re-identification refers to the task of finding the same subject across a network of surveillance cameras. This task must deal with appearance changes caused by variations in illumination, a person’s pose, camera viewing angle and background clutter. State-of-the-art approaches usually focus either on feature modeling – designing image descriptors that are robust to changes in imaging conditions, or dissimilarity functions – learning effective metrics to compare images from different cameras. Typically, with novel deep architectures both approaches can be merged into a single end-to-end training, but to become effective, this requires annotating thousands of subjects in each camera pair. Unlike standard CNN-based approaches, we introduce a spatial pyramid-like structure to the image and learn CNNs for image sub-regions at different scales. When training a CNN using only image sub-regions, we force the model to recognize not only the person’s identity but also the spatial location of the sub-region. This results in highly effective feature representations, which when combined with Mahalanobis-like metric learning significantly outperform state-of-the-art approaches.
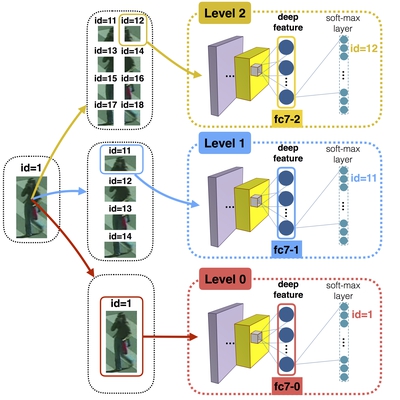