Local Anatomically – Constrained Facial Performance Retargeting
We present a new method for high-fidelity offline facial performance retargeting that is neither expensive nor artifact-prone.
July 24, 2022
ACM SIGGRAPH 2022
Authors
Prashanth Chandran (DisneyResearch|Studios/ETH Joint PhD)
Loïc Ciccone (DisneyResearch|Studios)
Markus Gross (DisneyResearch|Studios/ETH)
Derek Bradley (DisneyResearch|Studios)
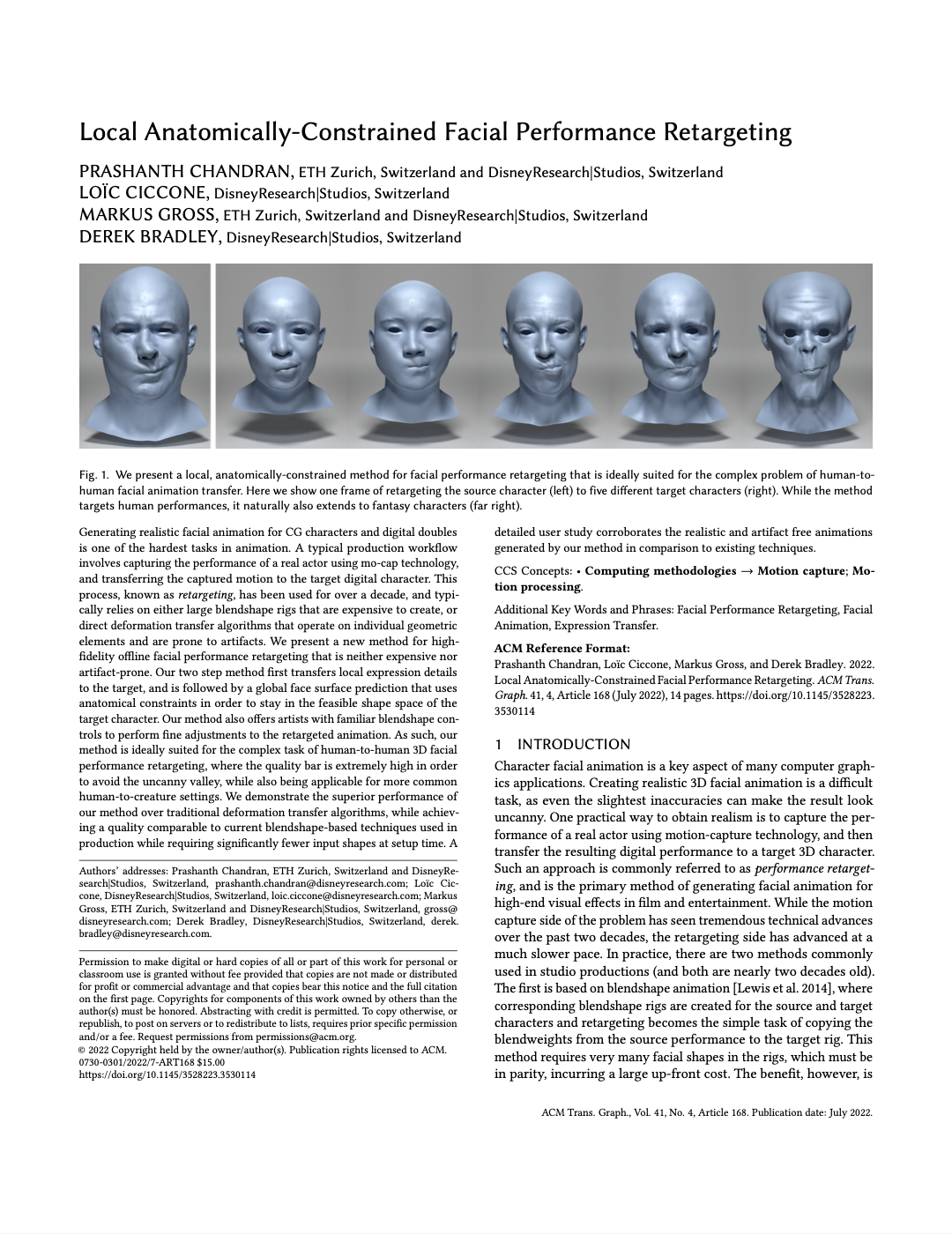
Local Anatomically – Constrained Facial Performance Retargeting
We present a new method for high-fidelity offline facial performance retargeting that is neither expensive nor artifact-prone. Our two-step method first transfers local expression details to the target and is followed by a global face surface prediction that uses anatomical constraints in order to stay in the feasible shape space of the target character. Our method further offers artists with familiar blendshape based controls to perform fine adjustments to the retargeted animation. As such, our method is ideally suited for the complex task of human-to-human 3D facial performance retargeting, where the quality bar is extremely high in order to avoid the uncanny valley.
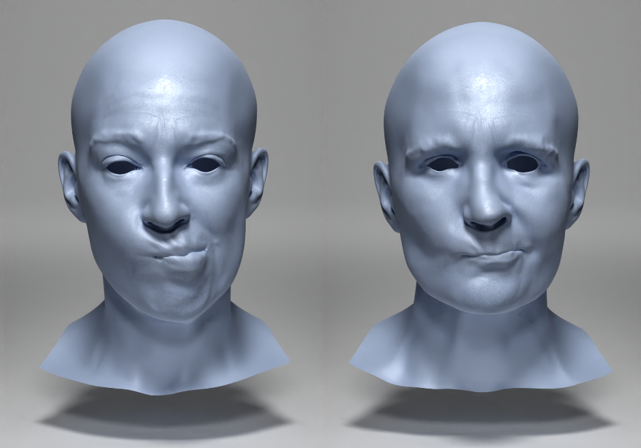