Contrastive Learning for Controllable Blind Video Restoration
We demonstrate state of the art results compared to most recent video super-resolution and denoising methods.
November 21, 2022
British Machine Vision Conference (BMVC) (2022)
Authors
Givi Meishvili (DisneyResearch|Studios)
Abdelaziz Djelouah (DisneyResearch|Studios)
Sally Hattori (The Walt Disney Company)
Christopher Schroers (DisneyResearch|Studios)
A lot of progress has been made since the first neural network models trained for specific image restoration tasks, such as super-resolution and denoising. Recently multi-degradation models have been proposed, allowing for user control of the restoration process which is needed for real world applications. This aspect is however most powerful if the initial restoration can be done as best as possible in a blind setting. In parallel to this line of works, other methods are able to target the blind setting where, for example in the case of super-resolution, the blur kernel is estimated for conditioning the restoration part. In particular, discriminative learning has played a key-role in pushing state of the art. Still the learned representation cannot be interpreted or manipulated, and remains a black box that doesn’t offer any possibility for user guided correction. In this work, we address those issues through a representation learning pipeline that helps separate content from the degradation by reasoning on pairs of degraded patches. The degradation representation is used as conditioning for a video restoration model that can denoise and upscale to arbitrary resolutions as well remove film scratches. Finally, the learned representation can be mutated to fine tune the restoration results and both the denoising and deblurring levels can be modified. We demonstrate state of the art results when comparing to most recent video super-resolution and denoising methods.
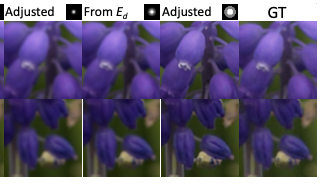