Efficient Video Encoder Autotuning via Offline Bayesian Optimization and Supervised Learning
We propose an efficient video encoder autotuner based on offline Bayesian optimization and supervised machine learning. Our proposal uses Bayesian optimization to search for a per-title best encoding parameter set offline to generate a dataset.
October 1, 2024
International Workshop on Multimedia Signal Processing (2024)
Authors
Roberto Azevedo (DisneyResearch|Studios)
Yuanyi Xue (Disney Entertainment/ESPN Tech.)
Xuewei Meng (Disney Entertainment/ESPN Tech.)
Wenhao Zhang (Disney Entertainment/ESPN Tech.)
Scott Labrozzi (Disney Entertainment/ESPN Tech.)
Christopher Schroers (DisneyResearch|Studios)
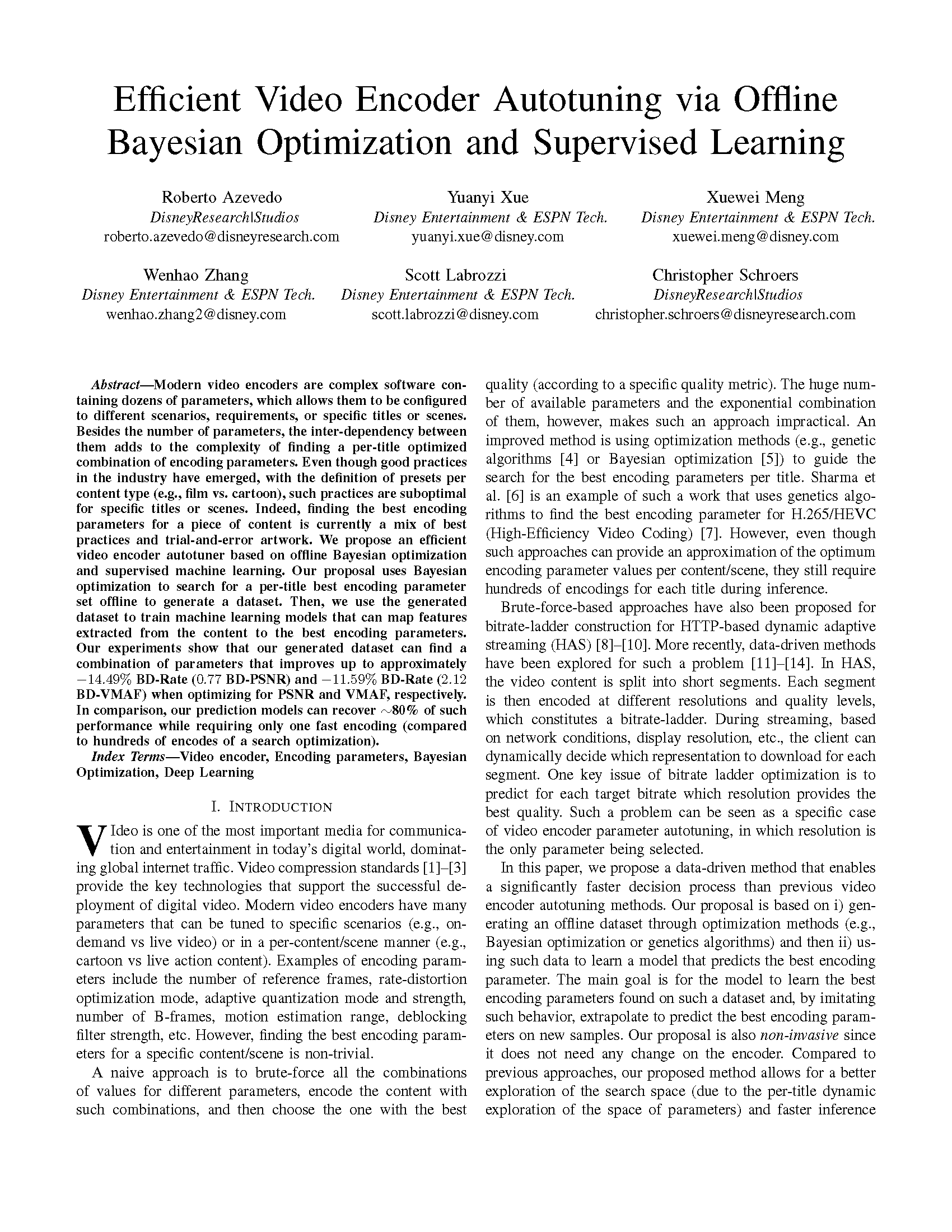
Efficient Video Encoder Autotuning via Offline Bayesian Optimization and Supervised Learning
Modern video encoders are complex software containing dozens of parameters, which allows them to be configured to different scenarios, requirements, or specific titles or scenes. Besides the number of parameters, the inter-dependency between them adds to the complexity of finding a per-title optimized combination of encoding parameters. Even though good practices in the industry have emerged, with the definition of presets per content type (e.g., film vs. cartoon), such practices are suboptimal for specific titles or scenes. Indeed, finding the best encoding parameters for a piece of content is currently a mix of best practices and trial-and-error artwork. We propose an efficient video encoder autotuner based on offline Bayesian optimization and supervised machine learning. Our proposal uses Bayesian optimization to search for a per-title best encoding parameter set offline to generate a dataset. Then, we use the generated dataset to train machine learning models that can map features extracted from the content to the best encoding parameters. Our experiments show that our generated dataset can find a combination of parameters that improves up to approximately −14.49% BD-Rate (0.77 BD-PSNR) and −11.59% BD-Rate (2.12 BD-VMAF) when optimizing for PSNR and VMAF, respectively. In comparison, our prediction models can recover ∼80% of such performance while requiring only one fast encoding (compared to hundreds of encodes of a search optimization).
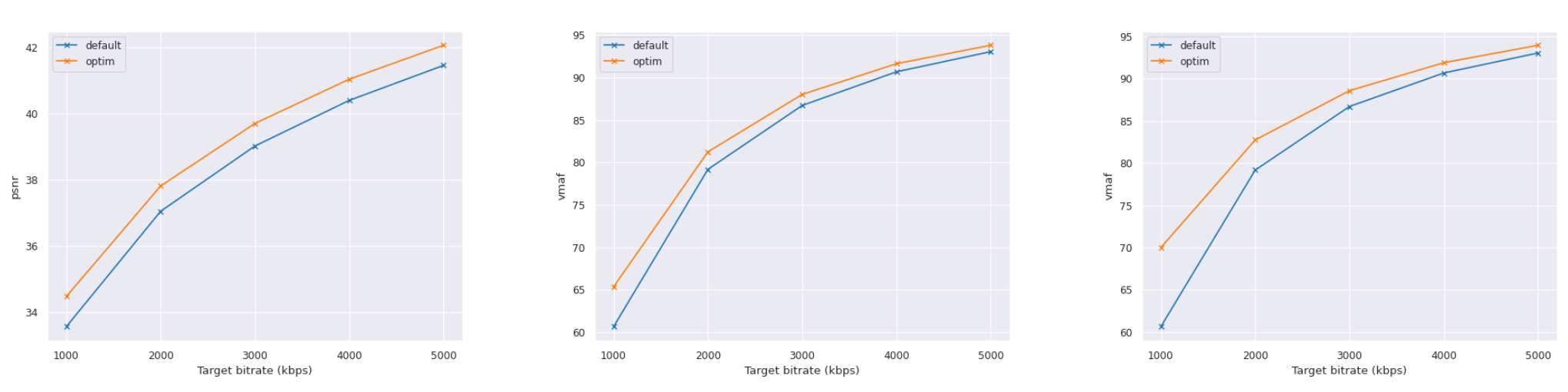