Revitalizing Legacy Video Content: Deinterlacing with Bidirectional Information Propagation
In this paper, we present a deep-learning-based method for deinterlacing animated and live-action content. Our proposed method supports bidirectional spatio-temporal information propagation across multiple scales to leverage information in both space and time.
November 24, 2024
British Machine Vision Conference (BMVC) (2024)
Authors
Zhaowei Gao (DisneyResearch|Studios / ETH Zürich)
Mingyang Song (DisneyResearch|Studios / ETH Zürich)
Christopher Schroers (DisneyResearch|Studios)
Yang Zhang (DisneyResearch|Studios)
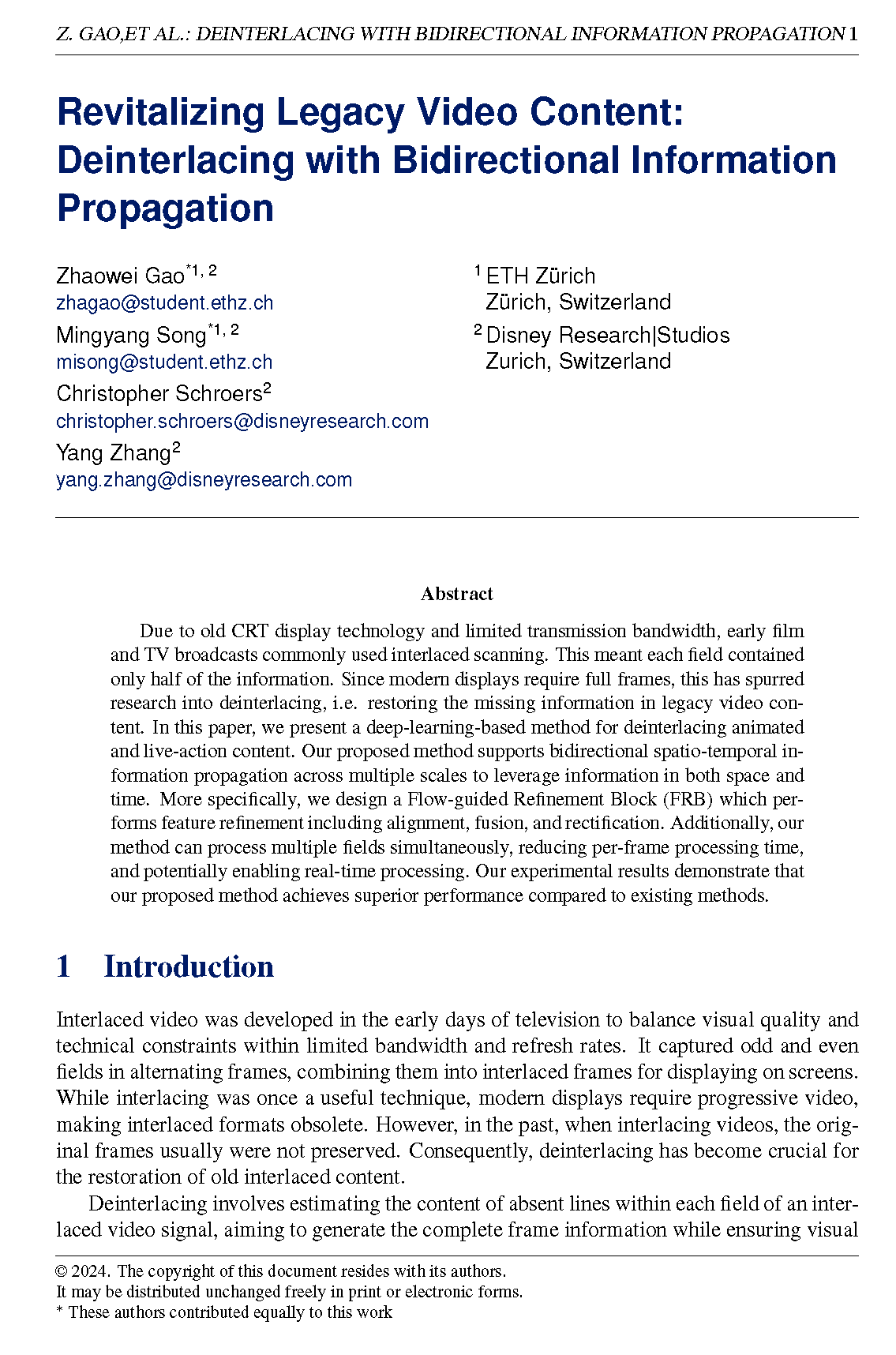
Revitalizing Legacy Video Content: Deinterlacing with Bidirectional Information Propagation
Due to old CRT display technology and limited transmission bandwidth, early film and TV broadcasts commonly used interlaced scanning. This meant each field contained only half of the information. Since modern displays require full frames, this has spurred research into deinterlacing, i.e. restoring the missing information in legacy video content. In this paper, we present a deep-learning-based method for deinterlacing animated and live-action content. Our proposed method supports bidirectional spatio-temporal information propagation across multiple scales to leverage information in both space and time. More specifically, we design a Flow-guided Refinement Block (FRB) which performs feature refinement including alignment, fusion, and rectification. Additionally, our method can process multiple fields simultaneously, reducing per-frame processing time, and potentially enabling real-time processing. Our experimental results demonstrate that our proposed method achieves superior performance compared to existing methods.
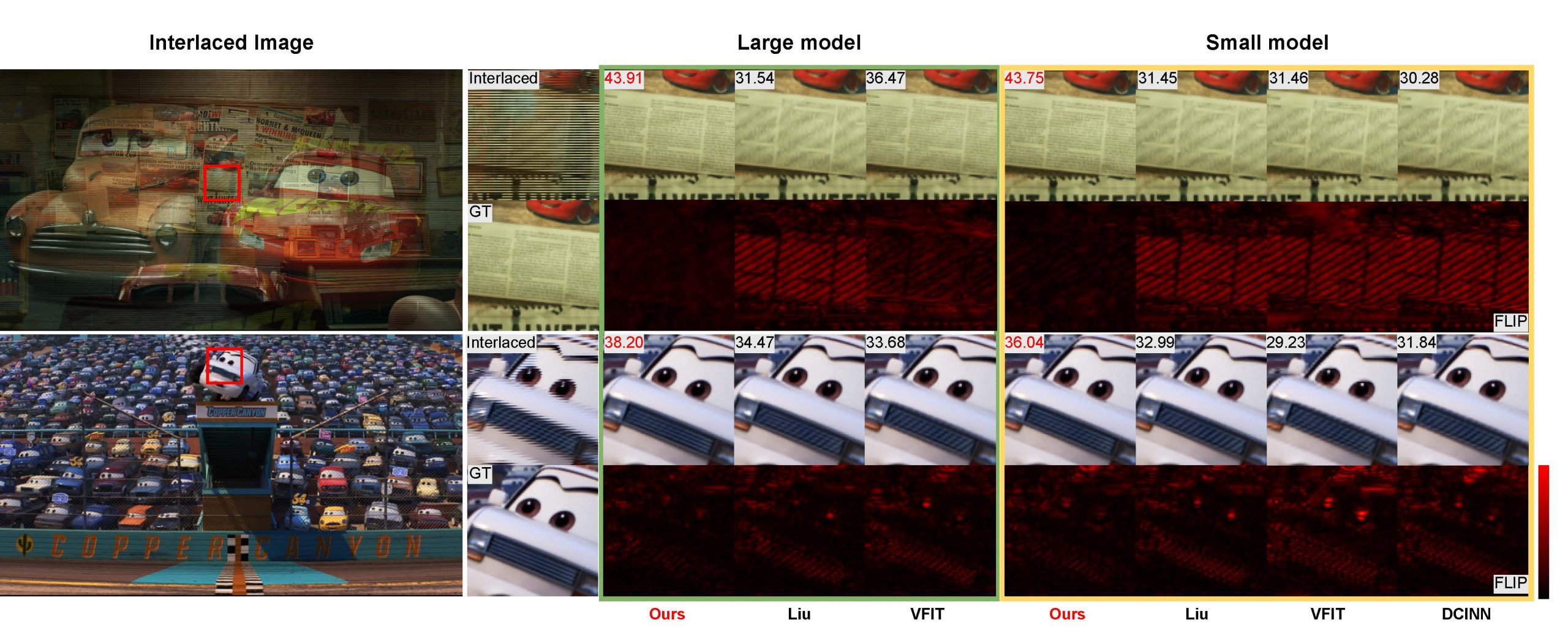