Smooth Imitation Learning
We study the problem of smooth imitation learning, where the goal is to train a policy that can imitate human behavior in a dynamic and continuous environment.
December 12, 2015
Neural Information Processing Systems (NIPS) 2015
Authors
Hoang M. Le (California Institute of Technology)
Yisong Yue (California Institute of Technology)
Peter Carr (Disney Research)

Smooth Imitation Learning
We study the problem of smooth imitation learning, where the goal is to train a policy that can imitate human behavior in a dynamic and continuous environment. Since such a policy will necessarily be imperfect, it should be able to smoothly recover from its mistakes. Our motivating application is training a policy to imitate an expert camera operator as she follows the action during a sport event; however our approach can be applied more generally as well. We take a learning reduction approach, where the problem of smooth imitation learning can be “reduced” to a regression problem, and the performance guarantee of the learned policy depends on the performance guarantee of the reduced regression problem (which is often much easier to analyze). Building upon previous learning reduction results, we can prove that our approach requires only a polynomial number of learning or exploration rounds before converging to a good policy. Our empirical validation confirms the efficacy and practical relevance of our approach.
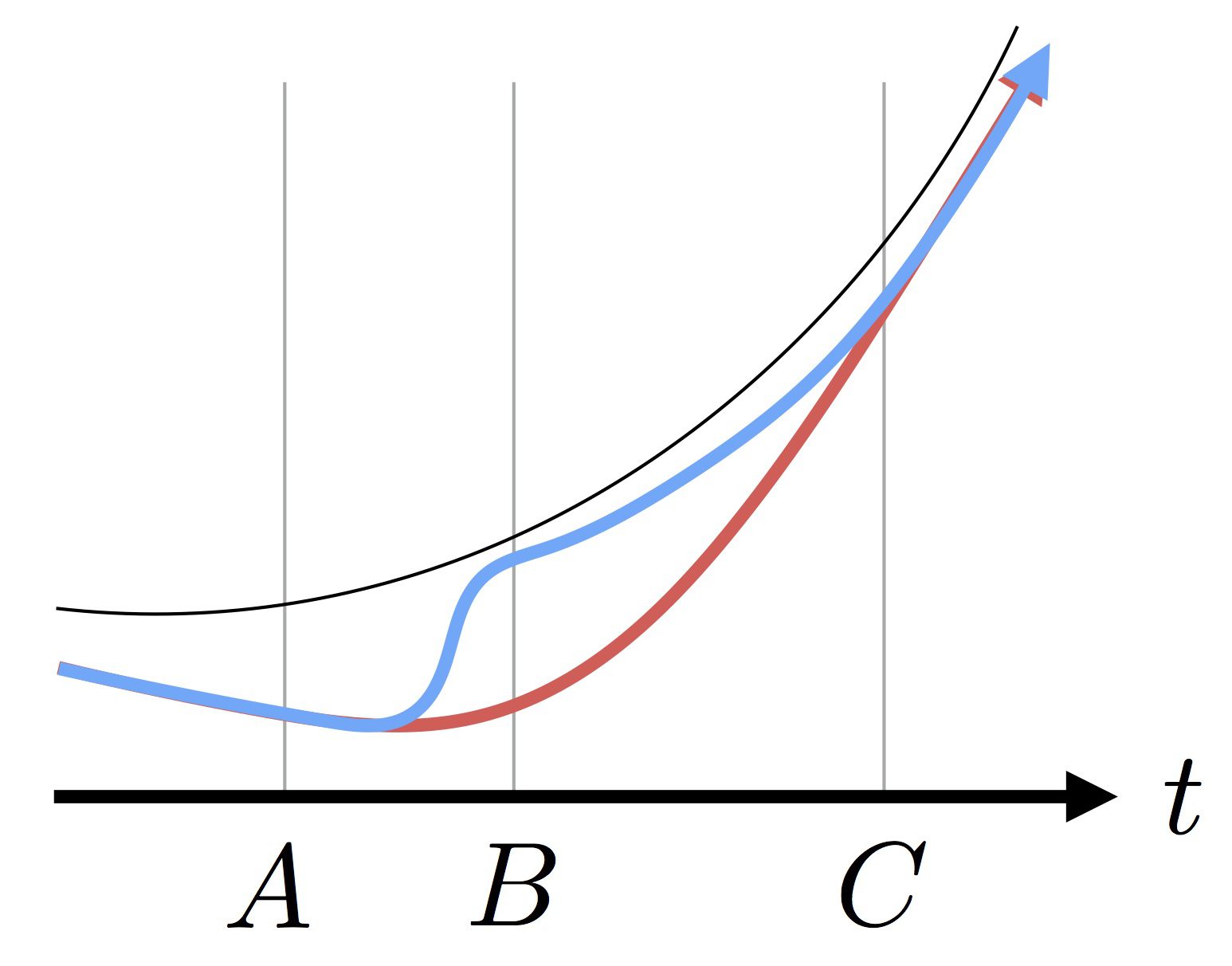