Exploiting View-Specific Appearance Similarities Across Classes for Zero-shot Pose Prediction: A Metric Learning Approach
We propose a metric learning approach for joint class prediction and pose estimation.
February 12, 2016
Association for the Advancement of Artificial Intelligence (AAAI) 2016
Authors
Alina Kuznetsova (Leibniz University Hannover)
Sung Ju Hwang (UNIST)
Bodo Rosenhahn (Leibniz University Hannover)
Leonid Sigal (Disney Research)
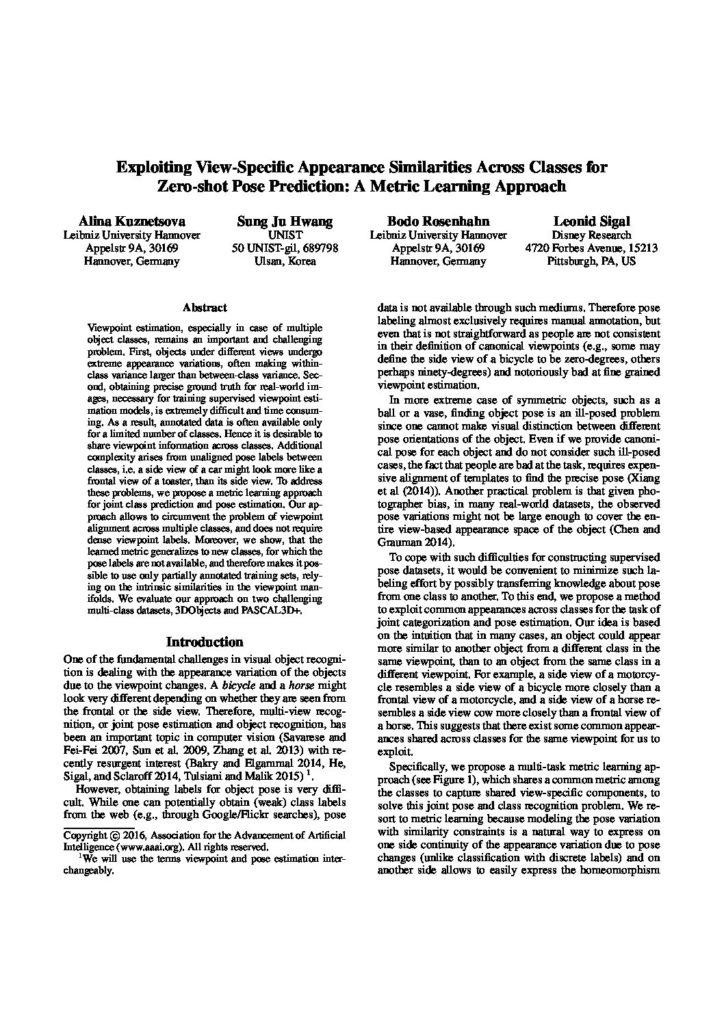
Exploiting View-Specific Appearance Similarities Across Classes for Zero-shot Pose Prediction: A Metric Learning Approach
Viewpoint estimation, especially in case of multiple ob- ject classes, remains an important and very challenging problem. First, objects under different views undergo extreme appearance variations, often making within- class variance larger than between-class variance. Sec- ond, obtaining precise ground truth for real-world im- ages, necessary for training supervised viewpoint es- timation models, is extremely difficult and time con- suming. As a result, annotated data is often available only for a limited number of classes. Hence it is de- sirable to share viewpoint information across classes. To address these problems, we propose a metric learn- ing approach for joint class prediction and pose estima- tion. Our metric learning approach allows us to circum- vent the problem of viewpoint alignment across multi- ple classes, and does not require precise or dense view- point labels. Moreover, we show, that the learned metric generalizes to new classes, for which the pose labels are not available, and therefore makes it possible to use only partially annotated training sets, relying on the intrin- sic similarities in the viewpoint manifolds. We evaluate our approach on two challenging multi-class datasets, 3DObjects and PASCAL3D+.
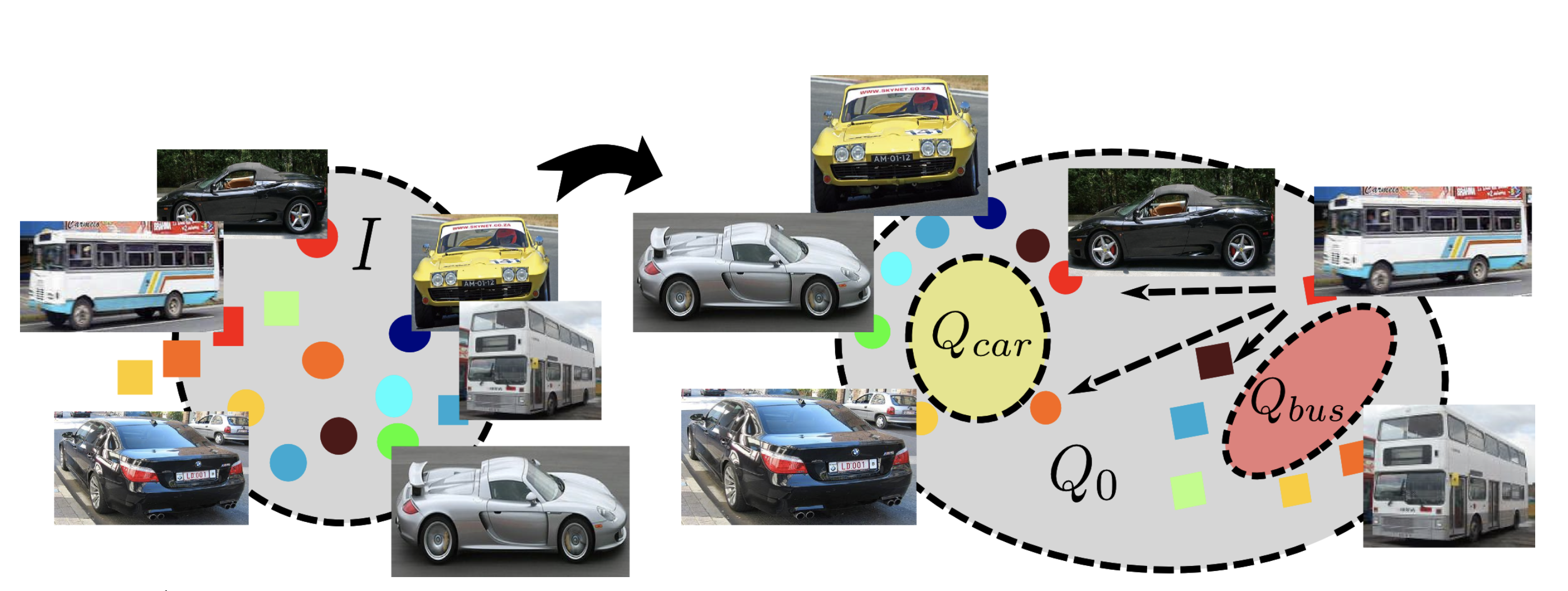