Suggesting Sounds for Images from Video Collections
In this paper, we aim to retrieve sounds corresponding to a query image.
October 8, 2016
ECCV Workshop (Computer Vision for Audio-Visual Media) 2016
Authors
Matthias Soler (Disney Research/ETH Joint M.Sc.)
Jean-Charles Bazin (Disney Research)
Oliver Wang (Disney Research)
Andreas Krause (ETH Zurich)
Alexander Sorkine-Hornung (Disney Research)
Suggesting Sounds for Images from Video Collections
Given a still image, humans can easily think of a sound associated with this image. For instance, people might associate the picture of a car with the sound of a car engine. In this paper, we aim to retrieve sounds corresponding to a query image. To solve this challenging task, our approach exploits the correlation between the audio and visual modalities in video collections. A major difficulty is the high amount of uncorrelated audio in the videos, i.e., audio that does not correspond to the main image content, such as voice-over, background music, added sound e ffects, or sounds originating o ff-screen. We present an unsupervised, clustering-based solution that is able to automatically separate correlated sounds from uncorrelated ones. The core algorithm is based on a joint audio-visual feature space, in which we perform iterated mutual kNN clustering in order to e ffectively filter out uncorrelated sounds. To this end, we also introduce a new dataset of correlated audio-visual data, on which we evaluate our approach and compare it to alternative solutions. Experiments show that our approach can successfully deal with a high amount of uncorrelated audio.
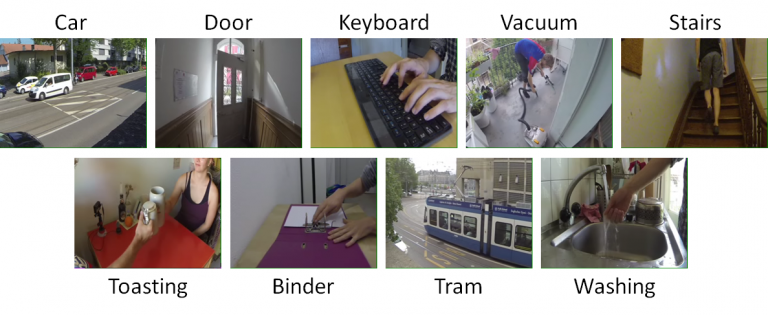