Efficient Neural Style Transfer For Volumetric Simulations
We propose a simple feed-forward neural network architecture that is able to infer view-independent stylizations that are three orders of the magnitude faster than its optimization-based counterpart.
November 30, 2022
ACM SIGGRAPH Asia (2022)
Authors
Joshua Aurand (DisneyResearch|Studios)
Raphaël Oritz (DisneyResearch|Studios)
Sylvia Nauer (DisneyResearch|Studios)
Vinicius Azevedo (DisneyResearch|Studios)
Artistically controlling fluids has always been a challenging task. Recently, volumetric Neural Style Transfer (NST) techniques have been used to artistically manipulate smoke simulation data with 2D images. In this work, we revisit previous volumetric NST techniques for smoke, proposing a suite of upgrades that enable stylizations that are significantly faster, simpler, more controllable and less prone to artifacts. Moreover, the energy minimization solved by previous methods is camera dependent. To avoid that, a computationally expensive iterative optimization performed for multiple views sampled around the original simulation is needed, which can take up to several minutes per frame. We propose a simple feed-forward neural network architecture that is able to infer view-independent stylizations that are three orders of the magnitude faster than its optimization-based counterpart.
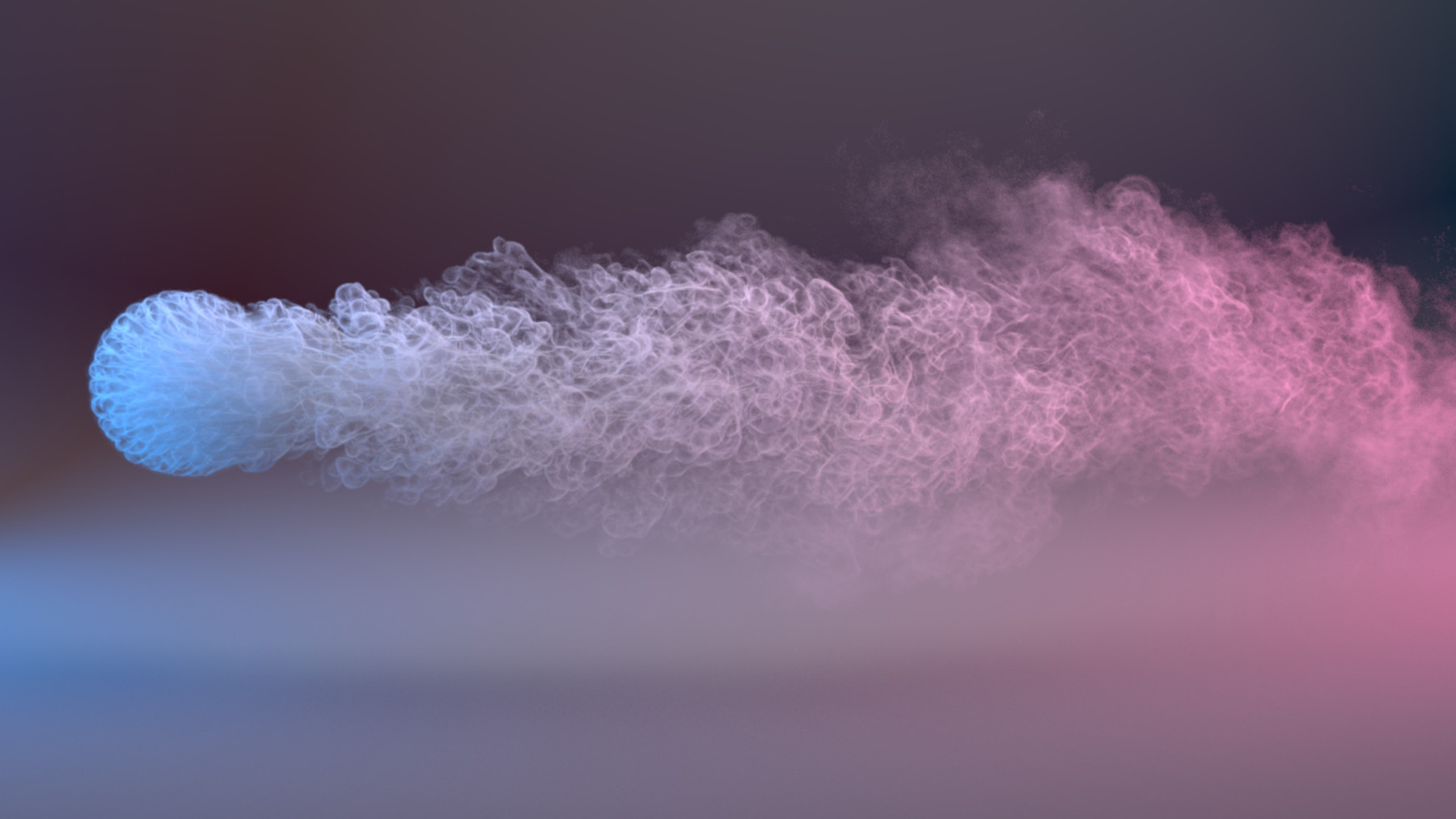